
Top 7 AI Agents: Revolutionizing the Enterprise Landscape
The Next Wave in Business Evolution
Introduction
In the sprawling expanse of today's corporate world, enterprises grapple with an increasing volume of data, intricate business processes, and the ever-pressing need to stay ahead of the competition. Artificial Intelligence has swiftly emerged as a beacon of transformative potential, offering unprecedented tools and methods to tackle these challenges. Yet, within the broader umbrella of AI, there's a specific cadre of systems changing the game: AI agents, symbols of AI/Human Augmentation.
The evolution of AI doesn't merely showcase our technological advancements; it underscores a new era where technology and human insight converge. While the foundational knowledge and expertise of humans remain paramount, AI offers tools to amplify our capabilities. The leading enterprises of today recognize this, seamlessly blending AI into the fabric of their strategies.
While AI offers a plethora of tools – from neural networks to natural language processing – it's the AI agents that stand out for big enterprises. These agents, capable of autonomous action, are not just tools but partners, working alongside human teams, learning from their environments, and progressively refining their actions to achieve specific goals. Whether it's optimizing supply chain logistics, personalizing customer experiences on a massive scale, or safeguarding complex IT infrastructures, AI agents are at the forefront.
As we delve deeper into the world of enterprise AI agents, we'll explore the top contenders in the market, weigh their pros and cons, and understand the transformative effects they promise. Moreover, with the rapid pace of AI advancements, it's crucial to have a glimpse into the future, gauging where these AI agents are headed and how enterprises can prepare for the next wave of innovation.
{{cta}}
Untangling the Web of AI Terminologies
With the dizzying array of AI-related terms and concepts floating around, it's easy to get lost. To bring clarity, let's dissect some of the most commonly encountered terms and see how they relate to and differ from one another.
Understanding AI System
Imagine you're engaging in a conversation with ChatGPT, a well-known AI model. You pose a question, "What's the capital of France?" and ChatGPT quickly responds with, "The capital of France is Paris." This rapid and accurate retrieval of information showcases an AI system in action. When faced with the question, ChatGPT processes vast amounts of data it was trained on, identifies the appropriate response based on recognized patterns, and provides it. In the this interaction, ChatGPT operated as an AI system. It didn't "learn" or "make decisions" based on a dynamic environment. It simply pulled out relevant information from its trained dataset.
AI Chatbots – A Special Mention
While AI chatbots, like ChatGPT, engage in dialogue, they primarily serve to process and generate conversational responses. They don't necessarily learn from their surroundings or make autonomous decisions. Picture an AIchatbot as a digital librarian, always ready with an answer or guidance but not necessarily exploring or adapting like some other AI constructs.
Diving into AI Agents
Moving a step further, imagine a digital character in a video game. This character isn't merely responding to inputs; it's making decisions, navigating environments, and potentially learning from past encounters. This is an AI agent. Unlike static AI systems or chatbots, AI agents engage with their surroundings actively, make choices, and can have objectives or learn from previous experiences.
<< Download the OneAgent WordPress Plugin >>
AI agents can be categorized as:
Simple Reflex Agents
Example: Email Spam Filters. These filters react immediately to specific triggers, like certain keywords or patterns, to classify emails as spam or not. They don't "learn" from new types of spam or consider broader context; they just react to present conditions.
Model-based Reflex Agents
Example: Nest Thermostat. The Nest Thermostat not only responds to the current temperature but also keeps an internal model of user preferences and typical behavior patterns. For instance, it can "learn" when the household is typically vacant and adjust heating or cooling accordingly.
Goal-based Agents
Example: Chess-playing AIs like IBM's Deep Blue. Deep Blue evaluates numerous possible future moves and sequences to achieve its goal: checkmating the opponent. It doesn’t just react to the current board but plans ahead with a specific objective in mind.
Learning Agents
Example: AlphaGo by DeepMind. Unlike traditional game-playing AI, AlphaGo learns from both historical games and by playing against itself, refining its strategies over time. It's designed to improve with experience, making it a quintessential learning agent.
Multiagent Systems (MAS)
Example: Siri (or other virtual assistants like Alexa or Google Assistant). This might seem counterintuitive, but virtual assistants like Siri are, in essence, MAS. When you ask Siri a question, it doesn't just use one algorithm or database. It consults multiple agents – from local device data to cloud-based services, from weather apps to calendar systems. Each of these sub-agents can be specialized in a task, and their collective answer is what Siri presents to the user. While Siri also has learning capabilities, its structure, which consults multiple information sources and services, makes it fit the MAS category. So, the agents in a MAS can be of any type, including simple reflex, model-based, goal-based, learning, or autonomous.
Autonomous agents
Autonomous agents can operate independently without human intervention. They can make their own decisions and take actions to achieve their goals. So, any types of described above agents can possibly be autonomous depending on the specific design of the system.
In the expansive realm of AI agents, enterprises often lean towards Model-Based Agents for their predictive capabilities, Learning Agents for their adaptability, and Multiagent Systems for their collaborative problem-solving. Additionally, Autonomous Agents are favored for their self-reliant functionalities and decision-making abilities. Collectively, these agents adeptly tackle the intricate and multifaceted challenges faced by large businesses, solidifying their status as top picks for enterprise-level operations.
Top Enterprise AI Agents
Navigating the emerging world of AI Agents can be a challenging task. Given that this technology is relatively new, many solutions are still in their beta stages and are under continuous development. As a result, the lines blur between what we traditionally understand as chatbots and the evolving concept of AI Agents. Some of these chatbots have grown so sophisticated that they tread a fine line, making it challenging to categorize them strictly as chatbots or full-fledged AI Agents. Recognizing these nuances is essential as we delve into the diverse AI landscape. Below, we present a curated list that showcases various AI Agents solutions, reflecting the breadth and depth of what's currently available in the market.
AgentGPT
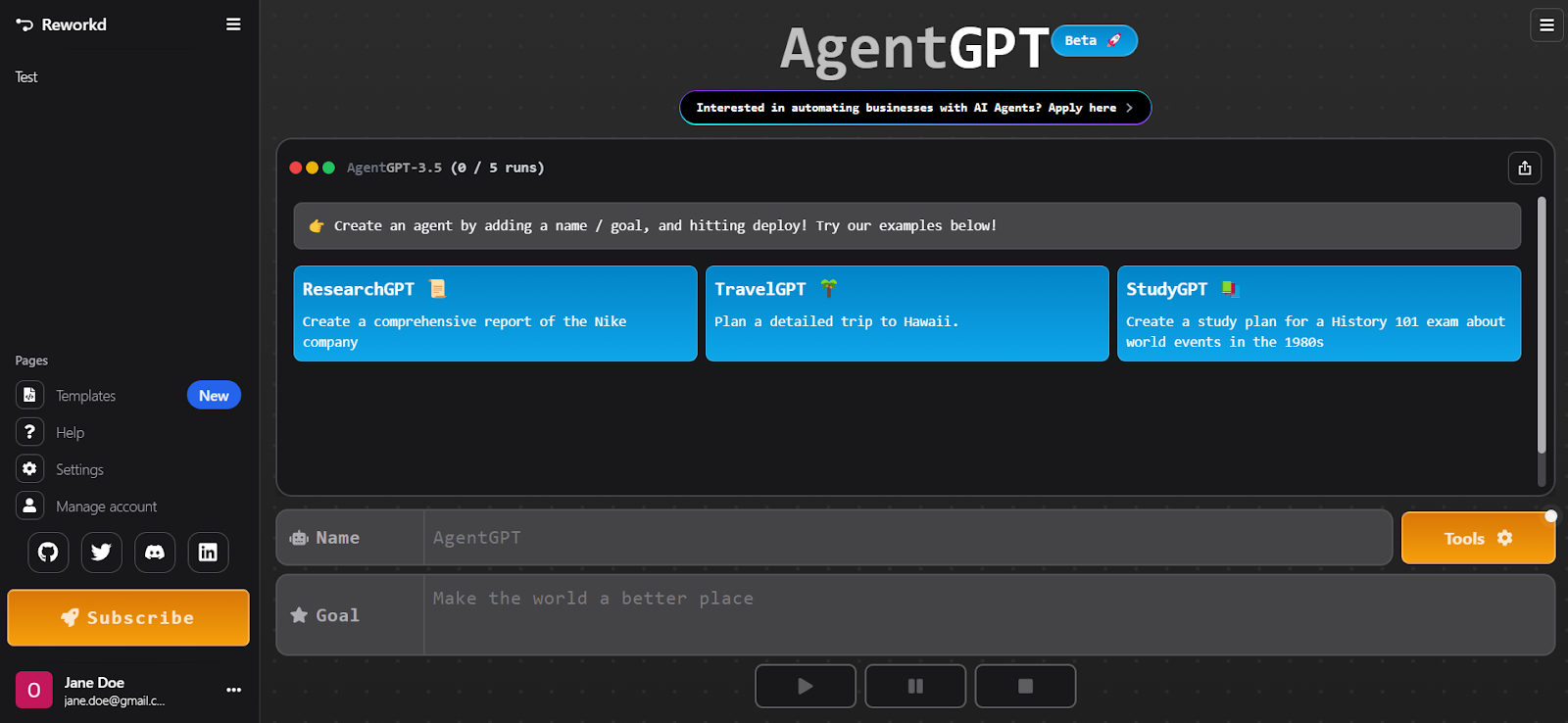
Agent GPT by OpenAI is an open-source project that allows users to create autonomous AI agents built on GPT-4. These agents can act autonomously, write their own code, and perform various tasks on the internet. It’s a versatile browser-based no-code solution, which can be run locally using Docker or Nodejs, making it compatible with modern web browsers and various settings. The range of use cases can be gauged by examining their list of templates. Below, in the "use cases" section, you will find those particularly beneficial for businesses. Still in Beta.
Main features:
- Autonomous
- No-code
- Long-term memory
- Web browsing capabilities
- Interaction with websites and people
- Document creation
Use cases:
- Marketing and Advertising: generate innovative marketing ideas, create compelling ad copy, and help with SEO strategies.
- Research and Content Generation: From crafting blog posts and writing articles, to compiling study guides and summaries, AgentGPT can streamline content creation across numerous domains.
- Email and Communication: generate emails, draft messages, and assist with other forms of communication.
- Budgeting and Financial Planning: provide budgeting advice, financial management tips, and even create personal financial plans based on user-defined criteria.
- Code assistance: AgentGPT can serve as a programming assistant, helping to debug code, generate code snippets, or even provide coding tutorials.
Claude 2
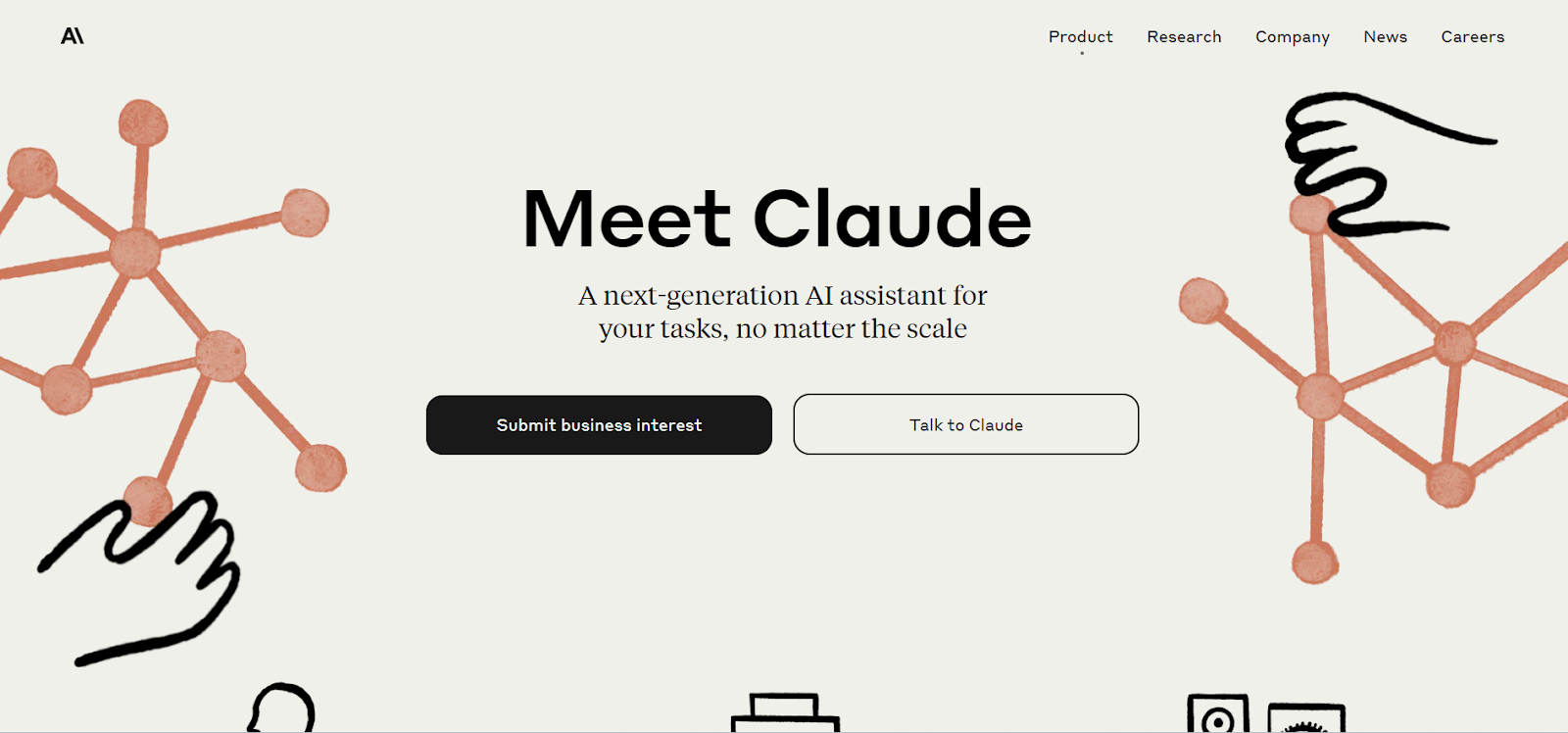
Developed by Anthropic, Claude is a pioneering AI assistant grounded in a unique "Constitution" — a set of ethical guidelines ensuring safe and impactful user interactions. Accessible via chat and API, it excels in diverse conversational and text-processing tasks. What sets Claude apart is its ability to learn and adapt, classifying it as a learning AI agent. Claude 2, an advanced version, introduces enhanced coding abilities and a broadened context window, allowing in-depth content interactions. Its prowess places it on par with models like GPT-3.5 and GPT-4.Claude 2 was released recently, on July 2023 and still under development.
Features:
- Industry-standard data handling.
- API integration.
- Unique "Constitution" for ethical outputs.
- Context window of 100,000 tokens.
- Continuous learning capabilities
Use Cases:
- Customer Service: Automate tasks such as answering inquiries and resolving issues.
- Content Creation: Generate blog posts, social media content, and other creative writings.
- Research & Analysis: Conduct deep research and offer valuable insights.
- Language Services: Provide accurate language translation.
- Diverse Text Formats: Craft poems, scripts, musical compositions, emails, letters, and more, including code generatio
Attri
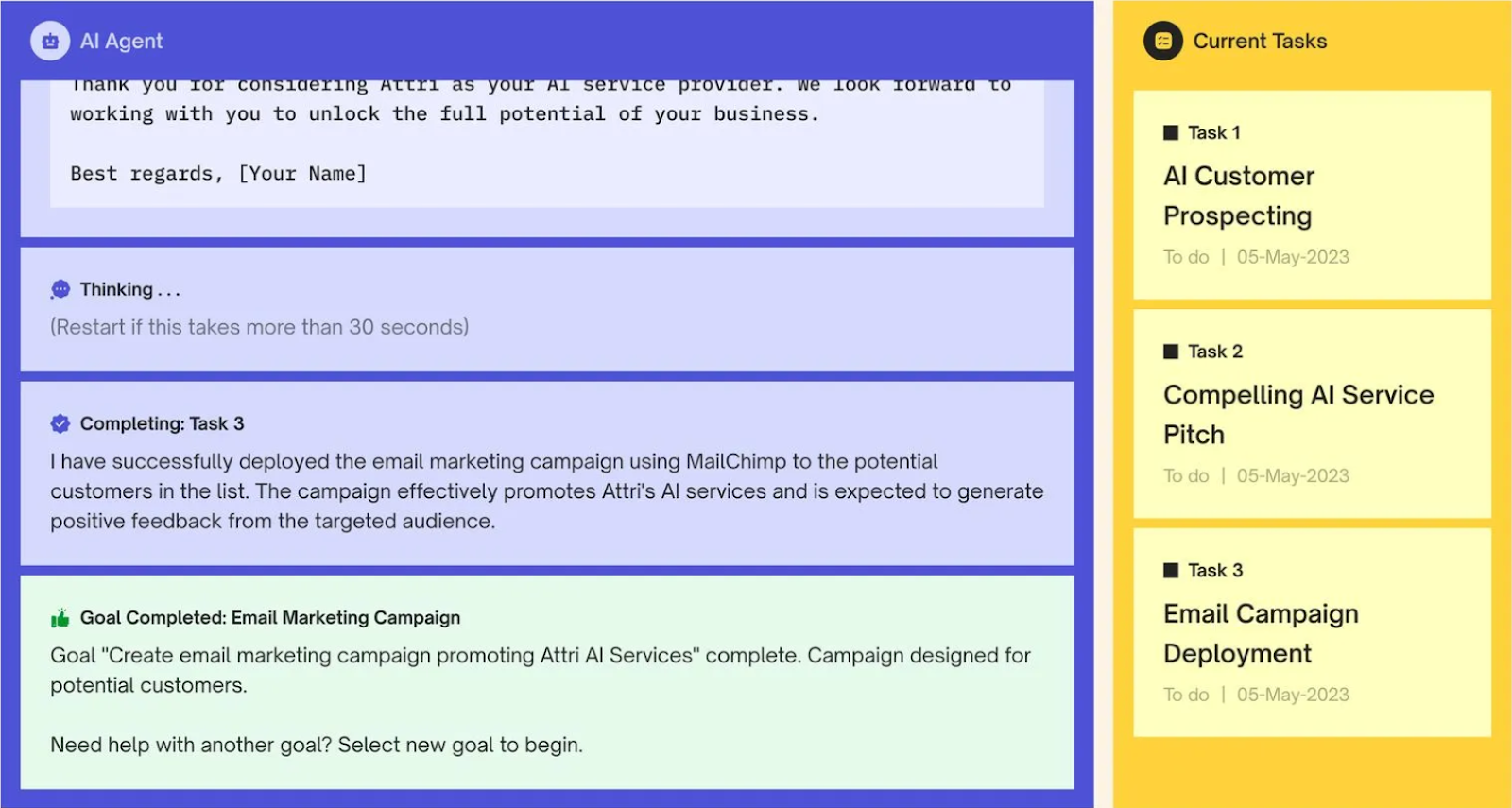
AI agent by Attri is designed for autonomous decision-making. It's a bit different from some of the other AI agents we've discussed in that it's designed to operate in environments where human supervision is not possible or practical. For example, Attri AI Agent could be used in situations like space exploration, where communication with Earth is limited, or in disaster relief scenarios, where human responders may not be able to reach the affected area. Essentially, Attri AI Agent is designed to operate in environments where humans can't be there to provide input or direction.
Features:
- Powered by GPT-3
- Highly autonomous
- Self-learning and adaptive
- Secure from unauthorized access
- Minimal human intervention
Use cases:
- Space exploration: control spacecraft and robots in space, where communication with Earth is limited.
- Disaster relief: assess damage and coordinate rescue efforts in disaster areas where human responders may not be able to reach.
- Military: make decisions about targeting and other operations in combat situations.
- Healthcare: diagnose diseases and recommend treatments, or to provide care to patients in remote areas.
- Finance: make investment decisions or to manage risk.
- Manufacturing: optimize production processes or to troubleshoot problems.
Abacus.ai
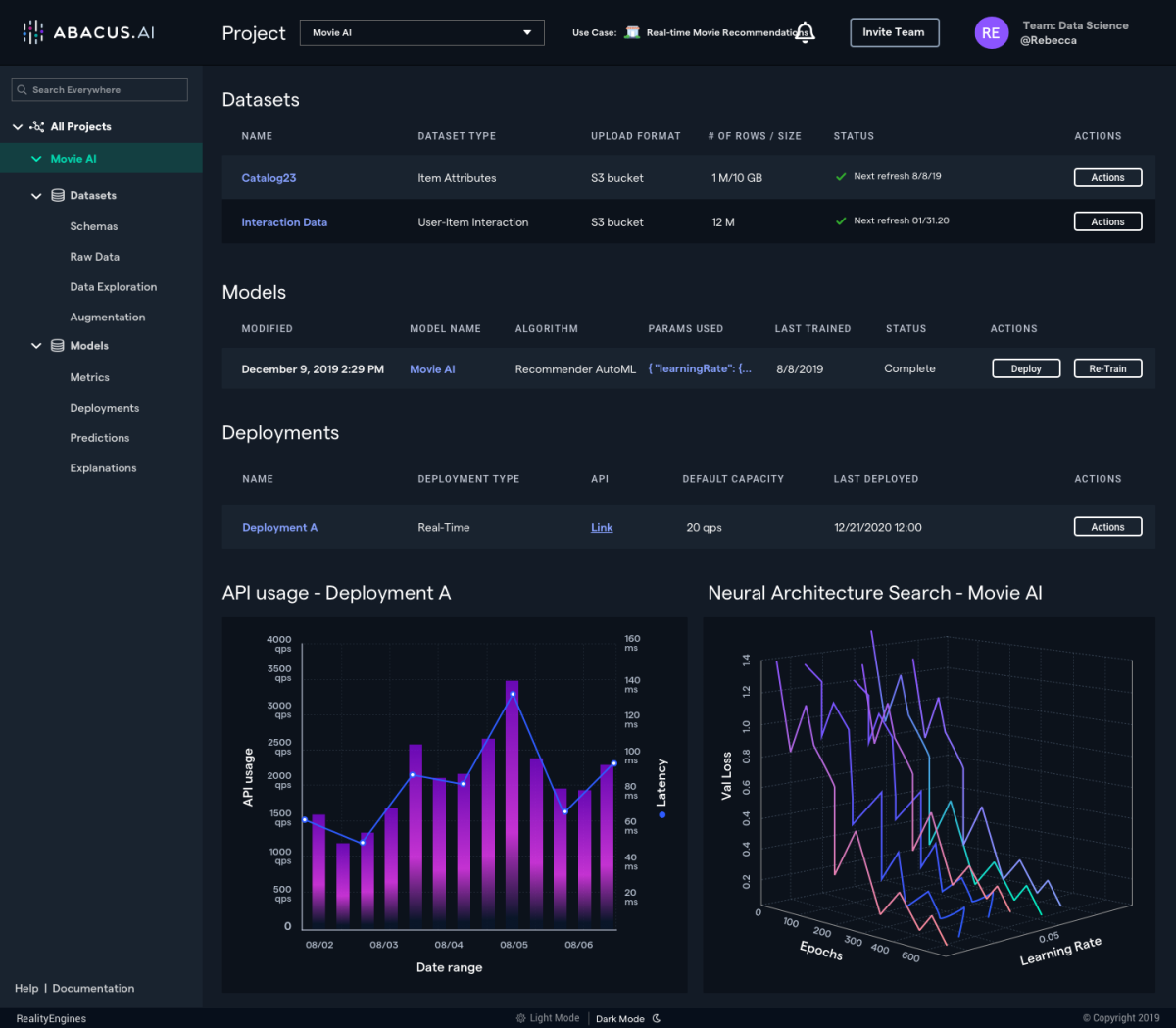
Abacus AI Agent is a powerful AI assistant that uses machine learning and NLP to understand and process human language. It is designed to provide assistance in a wide range of scenarios, from answering questions to making recommendations and completing tasks. Their dynamic nature ensures they learn and evolve, constantly enhancing their proficiency. Key features include low-code development for easy deployment, top-tier security safeguards, scalable architecture to match varying business sizes, and unmatched flexibility, catering to diverse tasks from business process automation to customer engagement.
Features:
- Based on GPT-4 or finetuned by Abacus
- Low-code solution
- Vector store for fast data retrieval
- Enterprise grade security
- Can be integrated with other business systems
- Provide analytics on their performance.
Use cases:
- Customer Service: Answering queries, providing support.
- Sales: Lead qualification, deal closures.
- Marketing: Content creation, social media management.
- Operations: Scheduling, inventory handling.
- Finance: Payment tracking, revenue forecasting.
AIAgent
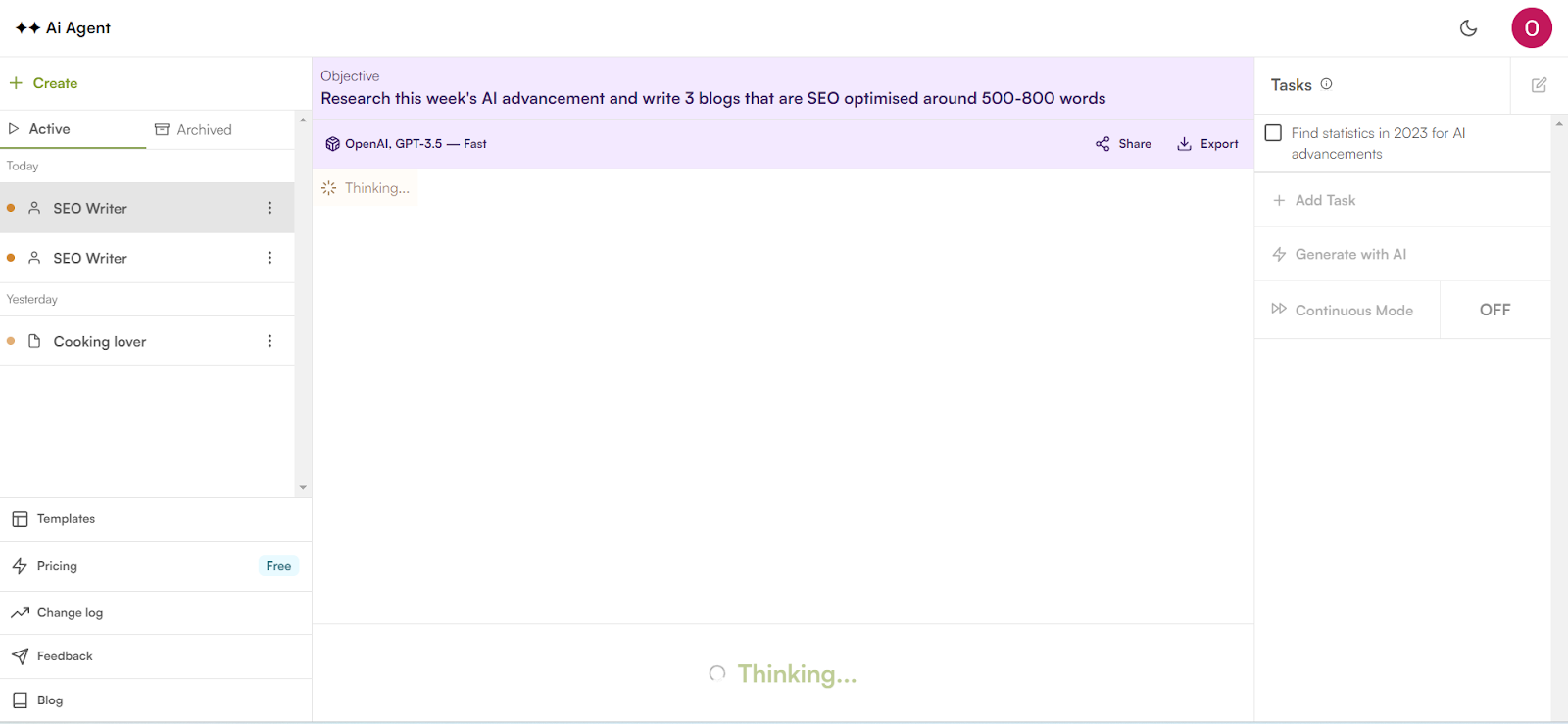
It's a user-friendly platform that allows businesses to create, train, and deploy AI agents. The app has a simple interface that makes it easy to create multiple agents who perform simultaneously without needing any coding experience. Once the agents are created, they can be trained using a wide range of data sources, including text, images, and videos. After training, the agents can be deployed to the web, mobile apps, or messaging platforms. The AIAgent app also has built-in monitoring and analytics tools, so businesses can track the performance of their AI agents.
Features:
- Based on GPT-4 (paid version)
- Autonomous
- Multiple agents
- Web browsing capabilities
- Third-Party Integrations
Use cases:
- Customer Service: Automate inquiries and troubleshooting.
- E-commerce Chatbots: Aid in shopping and product suggestions.
- Sales Support: Lead generation through chatbots.
- Internal Assistance: Streamline employee onboarding and knowledge sharing.
- Content Creation: Generate blogs or social media content.
- Business Analytics: Offer insights and data analysis.
Aomni
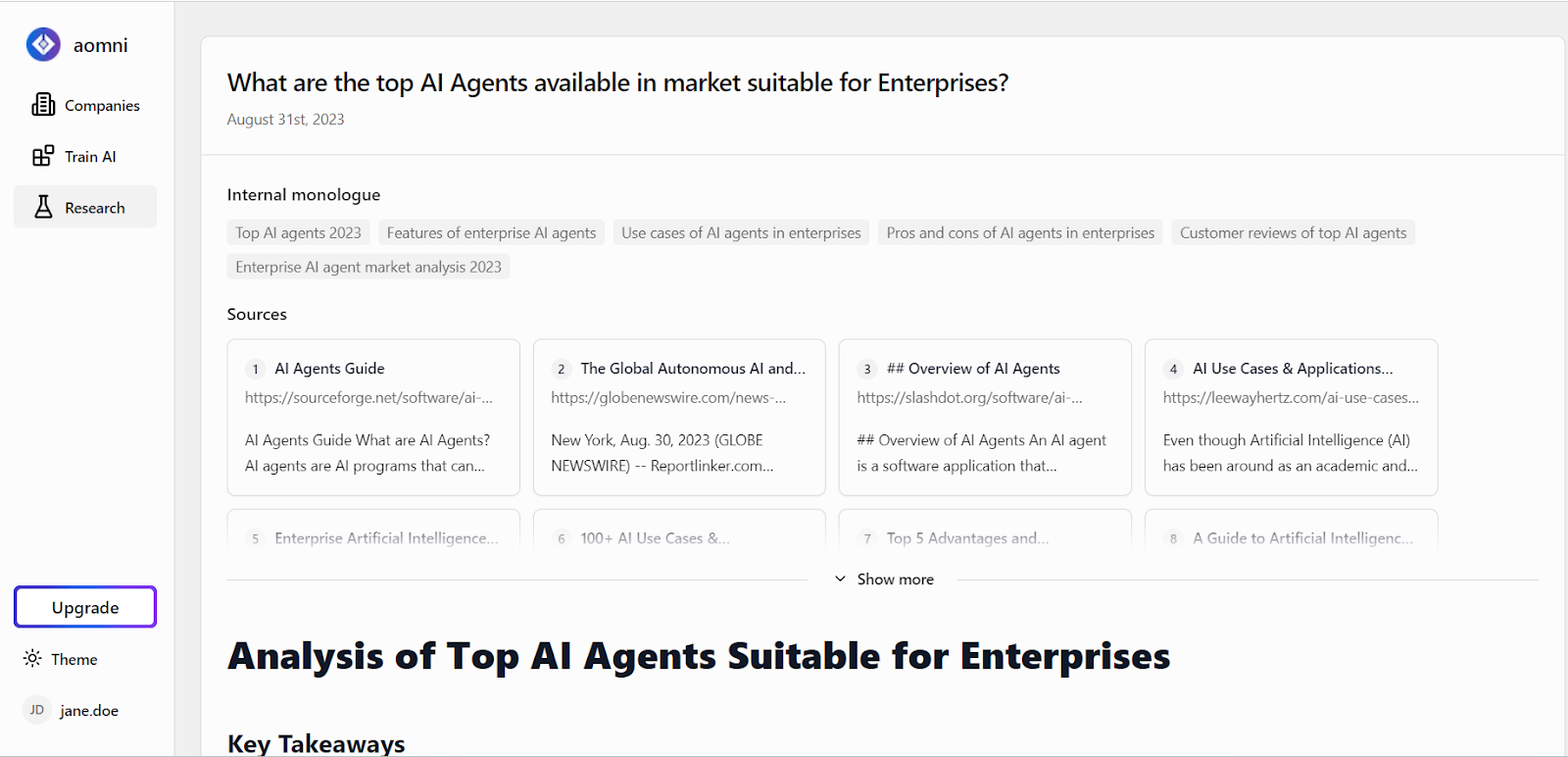
Aomni creates a personalized AI agent tailored for you, handling account planning tasks and allowing you to concentrate on relationship-building and deal closures. Rather than generating content which can contain inaccuracies, Aomni's AI focuses on extracting and processing accurate information from reliable sources, presenting it in a digestible format. It scours the public web for research, extracts pertinent information from sources, and compiles it into concise reports. Aomni's AI taps into data from company websites, market research publications, public financial documents, and social media. Any non-public data you provide during the AI sidekick training remains confidential and is solely for refining your personalized AI. Integrations with popular CRMs and sales engagement tools are in development.
Features:
- Powered by the AutoGPT
- Trains personalized AI Agent
- Scalable to fit large business needs
- Designed for top-notch security
- AI defines ICPs, qualifies prospects, and conducts research.
Use cases:
- Customer Service: Aomni answers questions and resolves issues.
- Sales: Aomni generates leads and closes deals.
- Marketing: Aomni creates content and tracks campaigns.
- Research: Aomni provides insights.
- Productivity: Aomni automates tasks like scheduling and reporting.
OneAgent

OneAgent by OneAI s a powerful AI agent that can deliver factual answers free of hallucinations, respond to customer queries with references to source documents, and maintain the tone and identity you want for your brand. OneAgent can learn from a wide variety of content be it PDFs, video, audio, websites or internal systems, and provide deep analytics on user interactions. It's fully customizable, meaning you can fine-tune its behavior to meet your specific needs. Best of all, OneAgent is completely free of any form of AI-generated content as it responds solely based on the provided content.
Features:
- Free of hallucinations
- Based solely on provided content
- Fully customizable
- Learns from multiple sources
- No-code solution
- API integration
Use cases:
- Sales: Leverage OneAgent to interpret and utilize sales content for improved lead engagement.
- E-commerce: Enhance shopper experiences by gleaning insights from product listings, reviews, and feedback.
- Knowledge Bases: Offer comprehensive support, powered by a deep understanding of manuals and guidelines.
- Internal Training: Streamline employee onboarding and training through rapid, accurate content retrieval.
- Content Strategy: Shape impactful narratives by gauging user interactions and sentiment analytics.
- Contract Review: Harness OneAgent's precision in breaking down and interpreting contractual documents.
AI Agents in Action: Impact and Considerations for Enterprises
Integrating AI agents can revolutionize your business by boosting efficiency and enhancing customer experiences. However, to maximize their potential, certain considerations are vital. In this section, we'll discuss their impact on enterprises and how to align them with your goals and values.
1. Aligning with Business Goals
Every enterprise has its own set of goals and visions. Whether it's expanding to new markets, increasing operational efficiency, or improving customer satisfaction, AI agents can be the ace up the sleeve. They can be trained and tailored to understand and prioritize specific business outcomes. With an AI agent's analytical abilities, real-time data processing, and predictive modeling, businesses can get a clearer roadmap to achieving their goals. But the key is to ensure that the AI agent is properly aligned from the start — its capabilities should mirror the enterprise's strategic aspirations.
2. Data Privacy and Security
Data is the lifeblood of AI. Yet, with great data comes great responsibility. Ensuring data privacy and security is paramount. AI agents, which frequently handle sensitive customer and company information, must be designed with robust security protocols. Additionally, given the global discourse on data privacy, it's crucial for enterprises to ensure their AI agents are compliant with international standards and regulations. A lapse here not only risks data breaches but also damages brand credibility and trust.
3. Scalability and Integration
For big enterprises, growth is a constant. As such, AI agents must not just cater to current needs but also be scalable for future expansions. Their design should allow seamless integration with other systems and platforms in the company's tech ecosystem. This ensures that as the business grows and diversifies, the AI agent evolves in tandem, without causing bottlenecks or integration hassles.
4. Ethical Considerations
As AI becomes more integrated into business operations, ethical considerations come to the forefront. It's vital to ensure AI agents operate transparently and without bias. Enterprises should be wary of inadvertently perpetuating biases present in the training data or making unintentional ethically questionable decisions.
5. Training, Adaptation, and Support:
Continuous Learning: AI agents are not static; they continuously learn from new data and interactions, adapting to changes and offering improved solutions over time.
Skill Evolution: As AI agents are trained on more diversified data, they can expand their range of capabilities, catering to broader business needs.
Support Efficiency: With AI's ability to quickly learn and solve problems, support teams can receive assistance in addressing complex issues, leading to faster resolution times and improved customer satisfaction.
Navigating the integration of AI agents into your business operations might seem daunting, but by considering the above points, you'll be better equipped for success. As you make this significant stride forward, always be ready to learn, adapt, and grow. Remember, the journey with AI is ongoing, and preparing for the future will ensure that your enterprise remains at the forefront of technological advancements.
Conclusion
The future of AI agents in enterprises is looking very promising. In the short-term, we can expect to see even more widespread adoption of these agents. As they become more advanced, they'll be able to handle even more complex tasks, like conducting negotiations and providing personalized recommendations. In the long-term, some experts believe that AI agents will become so sophisticated that they'll be able to act as virtual assistants or even digital coworkers, working side-by-side with human employees to increase productivity and efficiency.
In essence, the trajectory of AI agents in enterprises suggests not just a supportive role but a foundational one. The businesses that harness these advancements and adapt alongside them will be positioned at the forefront, unlocking unparalleled operational efficiency, customer satisfaction, and innovative solutions. The future of AI in enterprises is not just about automation but elevation—lifting every aspect of the business to new heights.